What is Data Matching?
Data Matching is the process of identifying and comparing records from different data sources to determine if they represent the same entity or share some level of similarity. The goal of matching data is to integrate and consolidate information from disparate datasets, improving data quality, accuracy, and consistency. This is particularly crucial in situations where data may be duplicated, incomplete, or inconsistent across various systems.
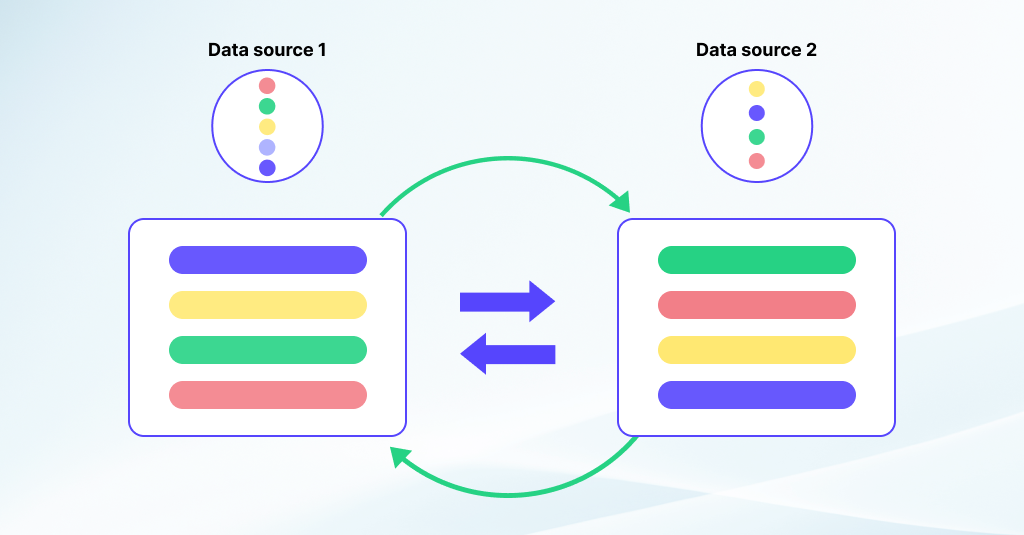
Key Aspects of Data Matching
Record Comparison: Data matching involves comparing individual records based on specific criteria, such as names, addresses, phone numbers, or other identifying attributes. The criteria used for comparison depend on the context and the nature of the data being matched.
Matching Algorithms: Matching algorithms are used to determine the similarity between records. These algorithms take into account variations, misspellings, and other discrepancies to identify potential matches. Common algorithms include exact matching, fuzzy matching, and phonetic matching.
Duplicate Detection: One of the primary purposes of matching data is to identify and eliminate duplicate records within a dataset. This helps prevent redundancy and ensures that data is accurate and up-to-date.
Data Cleansing: As part of the matching data, data cleansing may be performed to standardize and clean the data. This involves correcting errors, formatting inconsistencies, and normalizing data values.
Scoring and Thresholds: Matching algorithms often assign scores to pairs of records based on their similarity. A threshold is then applied to determine which pairs are considered matches. Adjusting the threshold can impact the trade-off between precision and recall in the matching process.
Entity Resolution: Entity resolution, or record linkage, is a broader concept that encompasses data matching. It involves linking records that refer to the same real-world entity, even if they don’t match exactly. This is crucial in scenarios where variations in data need to be reconciled.
Identity Management: Matching data is often used in identity management systems to ensure that a person or entity is correctly identified and represented across various databases or systems.
Privacy Considerations: When matching data, privacy considerations are important. Techniques such as anonymization or tokenization may be employed to protect sensitive information during the matching process.
Conclusion
Data matching is a critical step in the data integration and data quality improvement processes. It helps organizations maintain a unified and accurate view of their data, leading to more effective decision-making and improved operational efficiency.